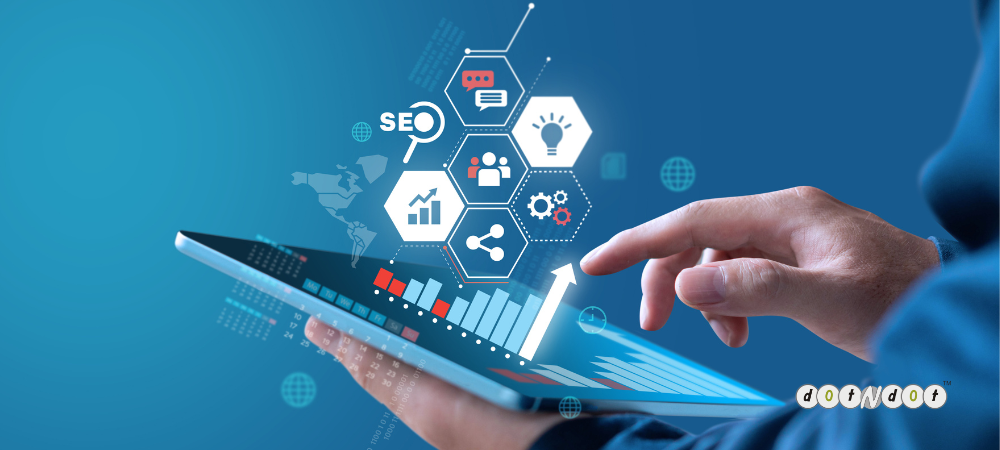
Market research is an essential aspect of any successful business venture. It is crucial to gather valuable insights into market trends and consumer preferences. But, sifting through massive amounts of data to identify relevant insights can be time-consuming and overwhelming.
This is where Principal Component Analysis (PCA) comes in. PCA is a powerful statistical tool that helps businesses make sense of large and complex datasets. By reducing the dimensionality of a dataset and extracting the most valuable information, PCA makes market research more targeted, efficient, and insightful.
We will dive into the world of market research with PCA, exploring how it works, its benefits, and real-world applications.
PCA is a statistical technique that reduces the complexity of a dataset by transforming it into a smaller set of uncorrelated variables known as principal components. These components represent the most significant sources of variance in the original dataset.
PCA identifies the linear combination of variables that explains the dataset’s most significant variance. It then uses these linear combinations to create principal components, each describing a decreasing amount of the conflict.
Introduction to Principal Component Analysis (PCA)
Principal Component Analysis (PCA) is a commonly used statistical technique widely applied in data analysis and machine learning tasks. The method works by transforming a dataset into a lower dimensional space to extract the underlying patterns and structure of the data.
This is achieved by finding the principal components and linear combinations of the original variables in the data that capture the most variance.
PCA is beneficial in situations where the original dataset contains many variables that may be highly correlated. By reducing the data’s dimensionality, PCA helps remove redundancy and noise while retaining the most vital information.
This can be particularly beneficial in image processing, genetics, and finance, where high-dimensional datasets are standard.
The History and Foundational Concepts of Principal Component Analysis
Principal Component Analysis (PCA) is a widely popular statistical technique used to transform a dataset with several variables into a smaller set of variables that can explain most of the variability in the original data. It was developed in 1901 by Karl Pearson as a method of simplifying complex datasets.
The foundational concept of PCA is to identify patterns within the data and use these patterns to create a more miniature representation of the original data that still accurately reflects the variations in the original dataset.
The primary goal of PCA is to reduce the number of dimensions of the dataset while preserving as much of the original information as possible. This makes it a handy tool in machine learning, data analysis, and image recognition.
Understanding Principal Component Analysis
Principal Component Analysis (PCA) is a statistical method used to reduce the dimensions of a high-dimensional dataset. It is a widely used technique in data science and machine learning, with applications in various fields such as finance, genomics, and image processing.
PCA aims to simplify the data by extracting the most significant patterns of variation from a dataset while retaining the maximum amount of information possible.
At a basic level, PCA works by constructing a new set of variables, linear combinations of the original variables in the dataset. These new variables, called principal components, are arranged in order of decreasing variance and represent the most significant sources of variation in the data.
The first principal component accounts for the most significant variation in the data, while the second main component accounts for the second-largest variation, and so on.
By projecting the data onto a reduced-dimensional space spanned by the first few principal components, we can effectively preserve the essential patterns of variation in the data while reducing its complexity.
The Importance of Principal Component Analysis in Market Research
Principal Component Analysis (PCA) is a well-known statistical technique that has gained significant attention in market research. This method plays a crucial role in analyzing data by reducing the complexity of the information while retaining its essential features.
PCA helps market researchers identify the most meaningful variables from a large data set and discover the data’s underlying patterns.
In Market Research, PCA can be utilized to analyze customer behavior, market trends, sales data, and consumer preferences.
By simplifying the data, PCA enables market researchers to identify and focus on critical factors that drive consumer behavior, which ultimately helps to create more efficient marketing strategies.
Exploring the Applications of Principal Component Analysis (PCA)
Principal component analysis (PCA) is a widely popular data analysis technique that has proven helpful in various applications. It is a statistical technique that uses linear algebra to reduce the dimensionality of the dataset by transforming a set of possibly correlated variables into a new set of uncorrelated variables called principal components.
PCA is a compelling method for data exploration, reduction of data redundancy, and noise reduction in the data set. PCA also finds large-scale structure in the data, making it an excellent tool for data visualization and cluster analysis.
One of the most common applications of PCA is in image processing and computer vision. PCA has been applied in facial recognition systems, where it is used to reduce the dimensionality of each image in a database of faces, making it easier and faster to compare and recognize them.
The same approach has been employed in medical imaging, where PCA has successfully reduced the dimensionality of the data, allowing for accurate visualization of medical images.
Discovering the Power of Principal Component Analysis in Multivariate Data Analysis
Principal Component Analysis (PCA) is one of the most potent techniques in multivariate data analysis. It is beneficial for analyzing complex data sets with many variables, as it reduces the dimensionality of the data while retaining important information.
PCA is a statistical method that transforms the original variables into a new set of variables, called principal components, which are linearly uncorrelated and account for the maximum variance of the data.
The main objective of PCA is to identify the underlying structure of the data by reducing the number of dimensions.
This is achieved by extracting the principal components and the directions along which the data varies the most. PCA is useful in data analysis because it can help reveal hidden patterns or correlations that might not be evident from the raw data.
How Principal Component Analysis (PCA) Reduces and Interprets Large Datasets
Principal Component Analysis (PCA) is a statistical technique that simplifies and interprets large datasets. PCA transforms a large dataset into a new set of variables, known as principal components, which capture the most significant information in the original dataset.
By reducing the dataset to smaller main pieces, PCA enables analysts and researchers to extract meaningful insights and interpret the data more efficiently.
PCA is beneficial when the relationship between variables is complex or difficult to discern. For example, in a dataset with multiple variables, it can be challenging to determine which variables are most significant and how they interact with each other.
PCA overcomes this challenge by identifying the variables that contribute most to the variation in the dataset and reducing the influence of variables that contribute less.
Exploring the Benefits of Principal Component Analysis in Market Research
Reduced Dimensionality
Principal Component Analysis (PCA) is a powerful tool used in market research that can help to reduce the dimensionality of data sets. PCA transforms a set of correlated variables into a new set of uncorrelated variables, known as principal components. This transformation helps reduce the number of variables while preserving most of the information in the original data set.
Improved Model Performance
PCA can also improve the performance of predictive models, such as regression or classification algorithms. PCA can reduce overfitting and improve model accuracy by reducing the number of dimensions in the data set. PCA can also identify essential features in the data set that may be useful for predicting outcomes or making decisions.
Enhanced Visualization
PCA can also enhance visualization techniques such as scatter plots and heat maps. Reducing the number of dimensions makes it easier to create visualizations that clearly show patterns and relationships between different variables in the data set. This makes it easier for market researchers to identify trends and insights that may have yet to be apparent before using PCA.
Improved Data Interpretation
Another benefit of using PCA is improved data interpretation. By transforming many correlated variables into smaller uncorrelated principal components, it becomes much easier to interpret and understand how different factors are related within a given market research study.
Applying Principal Component Analysis to Reduce Dimensionality and Uncover Patterns
Principal Component Analysis (PCA) is a commonly used statistical technique that has proven helpful in identifying hidden patterns and reducing the dimensionality of large data sets.
This method involves transforming a large set of variables into a smaller group of variables, known as principal components, that retain most of the information contained in the original data.
PCA can be used in various applications, from financial analysis to image recognition. In finance, for instance, PCA has been employed to analyze stock portfolios and extract the most important variables that help predict market trends.
In image recognition, PCA is utilized to identify groups of pixels exhibiting similar patterns, which can cluster images into different categories.
Utilizing Principal Component Analysis (PCA) to Simplify Data Analysis in Market Research
Market research is an ever-evolving field critical for businesses of all sizes and industries. However, analyzing the vast amounts of data collected during market research can be daunting for even the most experienced researchers. This is where Principal Component Analysis (PCA) can be an invaluable tool.
PCA is a statistical technique that simplifies data analysis by reducing the number of variables in a dataset while retaining the most essential information.
This allows for a more efficient analysis of large and complex data sets, making it easier to identify patterns and trends. PCA can identify hidden relationships between variables that may not be apparent with traditional analysis techniques.
Conclusion:
PCA is a powerful statistical tool that businesses can use to extract valuable insights from complex datasets. PCA makes market research more targeted and efficient by simplifying data, identifying patterns and relationships, and improving decision-making.
It has real-world applications in segmentation analysis, brand perception analysis, price sensitivity analysis, and product portfolio analysis.
However, businesses must approach PCA with careful planning and execution to ensure valid and meaningful results. Using PCA, companies can unleash the power of market research and gain a competitive edge in the marketplace.
In conclusion, PCA is a valuable tool for market research, which can help businesses gain deep insights into their audience’s preferences, attitudes, and behaviors.
Businesses can make better-informed decisions that drive growth and profits by reducing complex data into more straightforward yet informative variables. So, the next time you need to conduct market research, try PCA and see how it can help you gain a competitive edge.